Rethinking Data Engagement: Harnessing AI through Effective Data Orchestration
AI Data Strategy AGI Data Orchestration May 13, 2024 2:00:00 PM Steven Muir-McCarey 4 min read

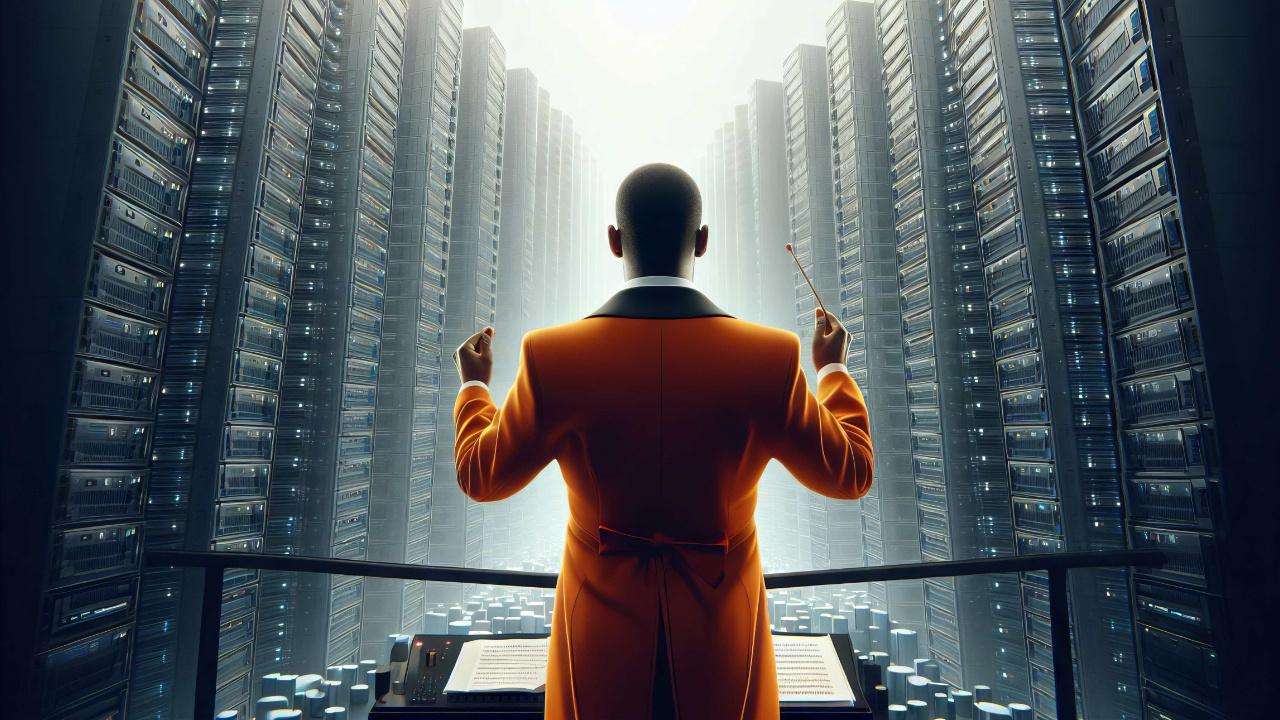
By Steven Muir-McCarey May 2024
As AI continues to reshape how we view and utilise data within organisations, engage publicly, and productise our offerings, mastering data orchestration has never been more critical.
Introduction
We believe that true adoption of AI within any business requires context. Context comes from organisational data, whether that is your customer, CRM, product, financial or marketing collateral. Whilst context is kind, surrendering data to these LLM's is a daunting proposition for even the most hardened and compliance-aware CxO's.
In this article we explore practical steps for delivering AI with data your organisational data. Key takeaways include actionable insights on building a data-centric culture and aligning AI capabilities with robust data management practices to drive innovation and operational efficiency.
The paradigm shift you need you go through in data management
AI is not just transforming businesses; it's revolutionising how data is perceived and utilised across more lines of business inside an organisation. By providing contextual insights tailored to user needs, AI has the potential to enhance internal processes, accelerate decision-making, and automate customer interactions and associated productisation.
However, the effectiveness of AI is heavily contingent on the quality and accessibility of data, highlighting the importance of robust data governance and seamless integration. This need is magnified with tools like GPT-4o that work best when fed with real-time data processing to in turn deliver now mere 200ms of a second audible response to complex queries and conversations.
Significant challenges arise with data that resides in disparate systems, such as customer information spread across marketing, billing, and CRM solutions. This scenario often leads to conflicts about the 'source of truth' for customer data.
Organisations might try to resolve this by extracting data into separate stores or data lakes, CDP's etc, which can result in data becoming stale, silo'd, quickly irrelevant, or inaccurate when removed from its operational context. Hence, a model that orchestrates and streamlines data flow with a well-structured framework is not just beneficial but necessary.
Practical Steps for AI Data Orchestration
You should focus on:
-
Align AI with Business Strategy: Ensure AI initiatives are directly linked to a core business problem aligned to the broader organisational objectives, anchored by the strategic revenue-generating business goals. Just "doing AI" isn't a business strategy and a crawl, walk, run framework should be put in place. Core CX business problems can include;
- Content and Brand Governance
- Predictive analytics insights
- Survey and Customer Sentiment analysis
- Personalisation strategies
- Funnel optimisation
- Chat & Customer Services Assistants
-
Establish Robust Data Governance: Implement comprehensive governance practices that enforce data integrity, security, and compliance across all systems, ensuring responsible management and usage of data throughout the organisation.
- Organisational policies
- HR & People training
- Physical & IT endpoint security
- Data Loss Prevention strategies
- MFA & Zero-trust access
- Data cleansing & data augmentation
- Master Data Management strategy
-
Build and Maintain Scalable Data Pipelines: Design data pipelines that are not only scalable but also optimised for AI processing, ensuring they can handle increasing volumes and complexities of data efficiently. This involves incorporating advanced data processing technologies and methodologies to support dynamic AI applications.
- Assess your data pipeline foundations
- Audit and assess existing database technologies
- Create capacity and scale plan
- Focus on data quality and health before scaling
-
Implement Continuous Feedback Loops: Establish mechanisms to continuously monitor, analyse, and iteratively improve AI models and data processes. This ensures ongoing refinement of AI systems based on performance feedback and evolving business needs.
- Consider cross-platform observability techniques
- Implement manual stage gates in your roadmap
- Align all decisions back to S.M.A.R.T goals
Conclusion
Mastering data fundamentals through effective orchestration is a critical component in realising the transformative potential of AI within any organisation. By adopting a framework approach to data orchestration, businesses can unlock a better-structured and more performant approach to AI adoption.
The latest commercially available GPT-4o capabilities further emphasise the necessity for robust data management strategies to fully leverage the advancements in these AI tools.
What Next?
Evaluating your organisation’s current AI approach & data management strategies? Consider the role of data orchestration in your holistic data strategy. This could not only help provide the structure in how applications access, share, and stream data but also accelerate conversations around data ownership within lines of business and application owners.
STAY ACROSS INDUSTRY TRENDS